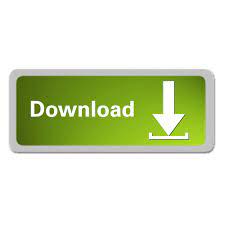
The bootstrap method employs a similar approach. If similar cluster solutions are obtained for graphs with some perturbation of edge-weights, this suggests the stability of the cluster solution for the original graph, providing the evidence of the community structure. A common approach to this problem is to evaluate the stability of cluster solutions when the data in question are noisy. Regarding statistical tests on community structure, several methods have been proposed in the context of unsigned (weighted or unweighted) graphs. In the present paper, we consider such a case. In this situation, it was shown that it is possible to detect community structures (in case of bisection) as n goes to ∞. In the context of unweighted graphs, this suggests that P a, b = c a, b. In the present paper, however, we focus on the case in which a generative model for edges has fixed parameters, irrespective of the number of nodes n. Further, it is shown that instead of a conventional adjacency matrix, a non-backtracking matrix, which represents non-backtracking walks in a network, provides a better platform for detection algorithms. In the situation in which an edge connection is generated by a probability P ab = c a, b/ n where c a, b is constant and n is the number of nodes, it has been shown that it is impossible for any algorithm to detect underlying community structure (as n → ∞) under certain circumstances. In the case of unweighted graphs, this issue has been intensively studied because of both mathematical and physical interest. For this framework, as a first step toward addressing a clustering problem, we aim to develop a statistical method for testing the existence of underlying community structure.įrom the theoretical point of view, there is the issue of detectability of community structure. Further, we assume that these distributions are distinguishable in terms of their mean and variance. We assume that edge weights are independently generated from a generative model that is specific to a particular cluster block, which characterizes a distribution of edges in each cluster block. Let us consider a general framework for community structure as follows. In the present paper, we address the question of community detection in a real-valued graph.
#Weighted standard deviation graph how to#
On the other hand, how to cluster nodes in a more general framework, such as negative edge weights within a cluster, remains an open question. Most methods in the literature, however, address this problem in a rather limited framework in which edge weights within a cluster are positive while those between clusters are negative (i.e., weakly balanced structure). Indeed, in real data, it is often essential to account for negative, as well as positive relationships, for a better understanding of the underlying community structure in a graph such as a social network. Recently, increased attention has been paid to analyzing signed graphs that allow negative weights. However, the conventional framework for analysis of community structure is typically an unsigned graph in which an edge weight is constrained to be non-negative. Panel (B): Matrix representation (edge-weight matrix), where strengths of relationships between nodes are denoted in color. Panel (A): Graphical representation (edge-weighted graph). Illustration of two-way community structure in a graph.
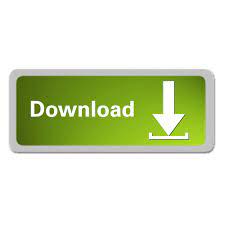